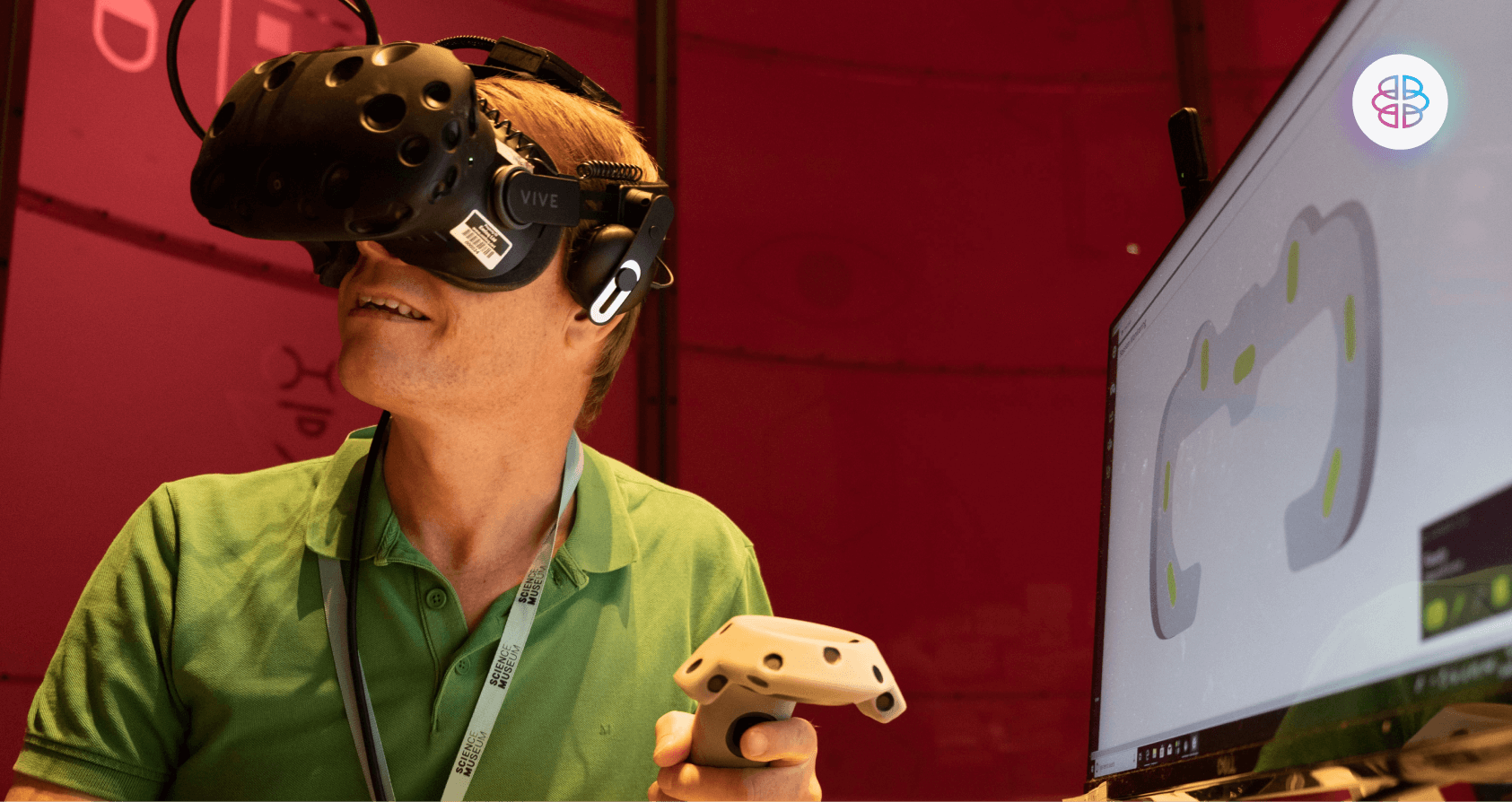
Understanding how people respond to their daily experiences is an important area of research. Insights from such studies have implications for understanding mental health, assessing responses to treatments and in the commercial settings (e.g. assessing responses digital or physical content).
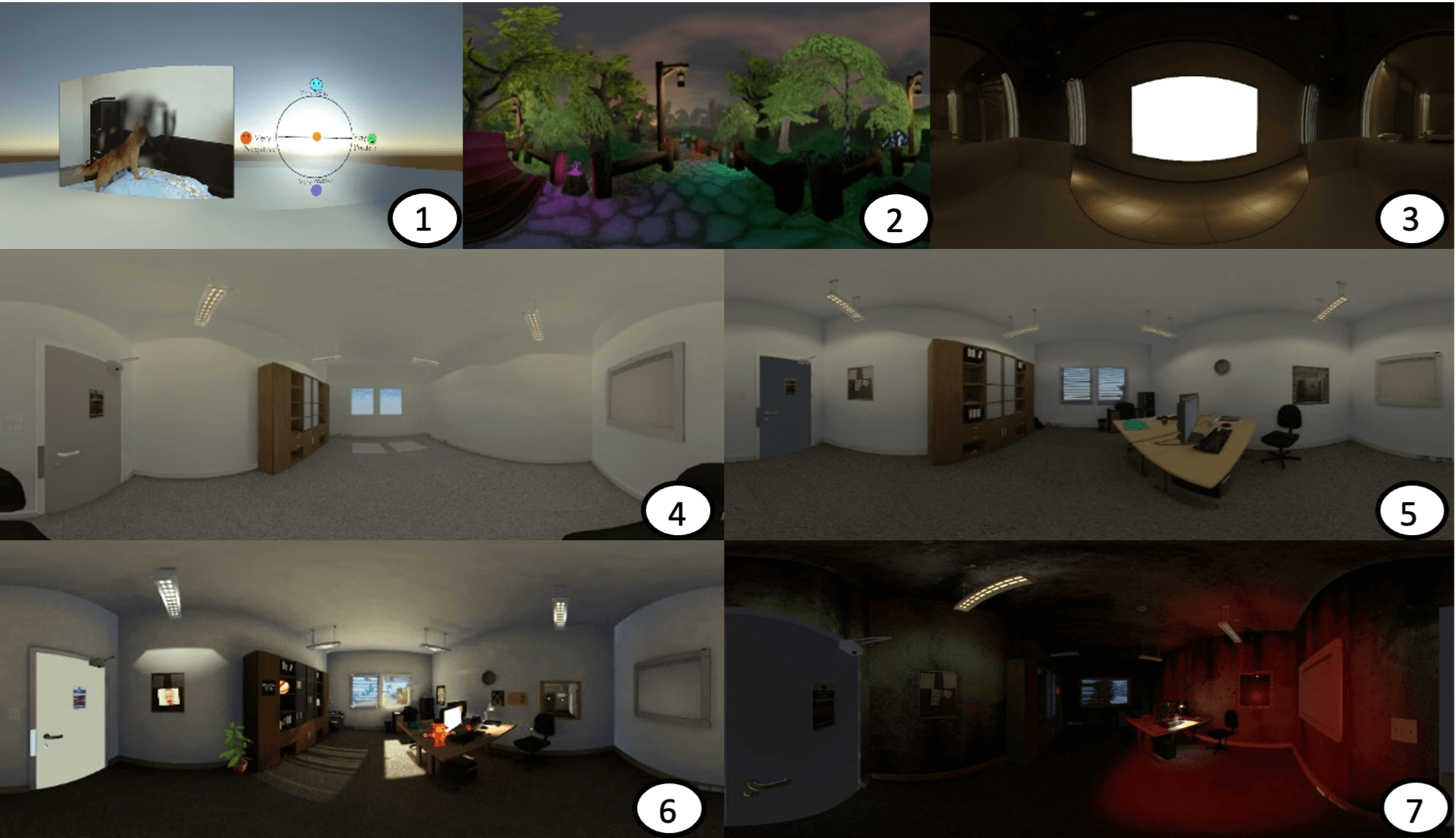
Until recently, researchers predominantly used non-immersive technologies such as TV/ PC screens to present content. In this study, Dr. Ifigeneia Mavridou and colleagues designed three experimental scenarios to test whether Virtual Reality (VR) affects how people respond to audiovisual content. The scenarios were based on an existing physical office, but with digital copies replicating the items in the virtual room.
The appearance and sounds made by the decorative props within this “digital twin” of the physical space were varied in their emotive qualities. To do this, the team created three versions of the items in the virtual environment (VE) corresponding to the emotional response being elicited. (valence and arousal) levels that it was indented to evoke (see figure 2). The selected stimuli were pre-evaluated by a group of participants via an online survey using videos and pictures (see AVEL library) An eye gaze-based interaction algorithm was implemented for the VR study, to record and mark the dynamic events throughout the participant’s experience with the immersive content. These events were then used as markers for the processing and analysis of the streamed data.

The VR experience had two modes of interaction: active and passive. In the active VR scenario, interactive stimuli were embedded within the 3-D environments and the participant was given complete control over her movement and duration of interaction with the stimuli surrounding her. In the passive scenario, participants’ overall interaction with the stimuli is constrained as they are presented via pre-recorded video.

The main study involved the execution of both experimental protocols (active and passive scenario) outside laboratory conditions at the Science Museum in London for six weeks. Approximately 730 datasets were recorded from visitors of the museum who volunteered to participate in the study. The recordings from each user derived from movement sensors, facial electromyography (EMG) sensors, heart-rate data from photoplethysmography (PPG) sensors and self-ratings (CASR) throughout the VR experiences.. Alongside sensor data collected during one’s experience with the content, participants’ information regarding their age, gender, current phobias, valence and arousal ratings per VE, memory scores per stimulus, personality traits, alexithymia levels and expressivity levels were collected using questionnaires. A comparison between the VEs and groups was conducted based on self-ratings and physiological signals.
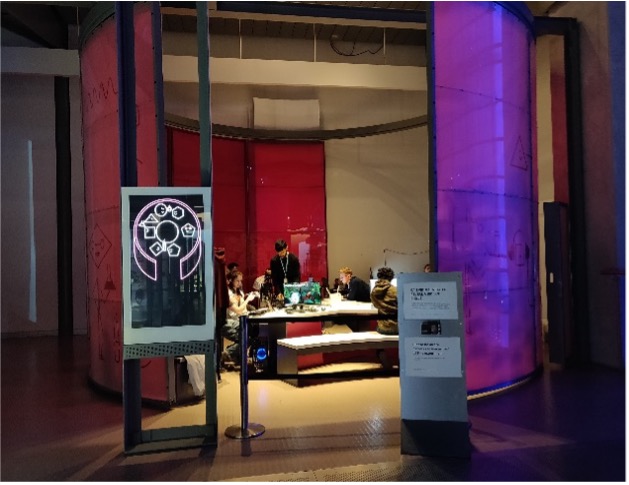
Taking advantage of the proposed affect detection system (explained in previous publications by the researcher), the relationship of the physiological data collected in VR with the self-ratings was explored. More particularly, the team was interested in utilising the physiological data collected to detect and potentially predict the affective ratings. For this reason, a set of features was extracted from the physiological sensors using time-domain metrics.
Selected findings/observation
· This study was one of the first VR studies to run in fully up-to-date immersive settings with free walking capabilities and achieved the collection of one of the largest biometric datasets outside laboratory settings. In other related studies, participants are recruited from predominantly student populations, while for this study a diverse population of highly motivated individuals participated.
· Overall, the two affective scenarios (positive and negative) elicited slightly higher intensities of arousal than the neutral scenarios. Their valence scores matched the expectations for each VE, regardless of the way the stimuli were presented to the participants (non-linear, interaction based, resulting to a different experience for each individual).
.png)
· The team also found that participants who actively experienced the VR environment (by freely walking and exploring) had a stronger emotional experience compared to the ones who watched the experience while seating on a chair (passive). Differences were found between the two groups as reflected in their self-ratings; the passive group was less susceptible to the affective manipulations, reporting overall lower arousal ratings than the active group, reduced memory accuracy scores, and reduced presence scores.
.png)
· Results for the analysis of the EMG signals show that spontaneous affect and specifically valence detection in VR settings can be reliable for both active and passive conditions. The dissociation between VE conditions was more pronounced on some channels for the active compared to the passive group.
.png)
.png)
· The heart-rate features extracted from the PPG sensor (IBI and rBPM) showed higher discriminatory power for the affective / high arousing VEs vs the low arousing, neutral VE condition for both groups, especially between the negative and the neutral VE condition.
.png)
· Notably, the continuous affect self-rating (CASR) method for self-rating was more sensitive than the single post-VE ratings, revealing additional difference between the two groups. See more information in the authors’ paper.
· The researchers utilised the data using machine learning approaches to classify three valence and arousal levels. The classification experiments yielded high accuracy results (between 80-92% accuracy based on the classifies and approach used) showing a good prediction potential for future applications. The highest accuracies were discovered when using personalised models (one model trained per dataset). This shows that future models could perform more robust if we follow a personalised model per user using data from previous sessions.
Concluding Remarks from the researchers:
“This is a very exciting output for the research community. Our finding s not only validate the use of the novel wearable sensors for continuous affect detection but also signify the feasibility of doing so within highly interactive VR settings that can induce naturalistic responses. Specifically, we were able to detect physiological arousal (the level of excitement or alertness) from heart-rate sensors and valence (how positive or negative an emotional state is) from EMG sensors that were integrated in the headset.
This opens new avenues for psycho-neuro-behavioural and cognitive research using VR. In future, it might be possible to utilize affective state detection as a core component for healthcare and wellbeing applications. As technological achievements continue to improve wearable sensors, we expect them to enhance and inspire further state detection using psychophysiological metrics outside strict laboratory conditions and in more naturalistic ways of interaction with stimuli, involving real and virtual situations.